Pearson's Correlation Coefficient: Deciphering the Strength and Direction of Linear Relationships
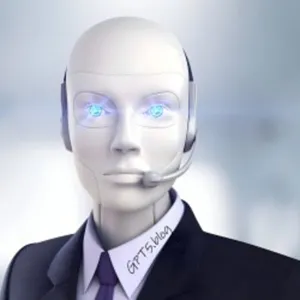
Pearson's Correlation Coefficient, denoted as r, is a statistical measure that quantifies the degree to which two variables linearly relate to each other. Developed by Karl Pearson at the turn of the 20th century, this coefficient is a foundational tool in both descriptive statistics and inferential statistics, providing insights into the nature of linear relationships across diverse fields, from psychology and finance to healthcare and social sciences
Key Characteristics and Applications
- Directionality: Pearson's r not only quantifies the strength but also the direction of the relationship, distinguishing between positive and negative associations.
- Quantitative Insight: It provides a single numerical value that summarizes the linear correlation between two variables, facilitating a clear and concise interpretation.
- Versatility: Pearson's correlation is used across a wide range of disciplines to explore and validate hypotheses about linear relationships, from examining the link between socioeconomic factors and health outcomes to analyzing financial market trends.
Calculating Pearson's Correlation Coefficient
The coefficient is calculated as the covariance of the two variables divided by the product of their standard deviations, effectively normalizing the covariance by the variability of each variable. This calculation ensures that r is dimensionless, providing a pure measure of correlation strength.
Considerations in Using Pearson's Correlation
- Linearity and Homoscedasticity: The accurate interpretation of r assumes that the relationship between the variables is linear and that the data exhibit homoscedasticity (constant variance).
- Outliers: Pearson's r can be sensitive to outliers, which can disproportionately influence the coefficient, leading to misleading interpretations.
- Causality: A significant Pearson's correlation does not imply causation. It merely indicates the extent of a linear relationship between two variables.
Limitations and Alternatives
While Pearson's correlation is a powerful tool for exploring linear relationships, it is not suited for analyzing non-linear relationships. In such cases, Spearman's rank correlation or Kendall's tau might be more appropriate, as these measures do not assume linearity.
Conclusion: A Pillar of Statistical Analysis
Pearson's Correlation Coefficient remains a central pillar in statistical analysis, offering a straightforward yet powerful method for exploring and quantifying linear relationships between variables. Its widespread application across various scientific and practical fields underscores its enduring value in uncovering and understanding the dynamics of linear associations.
Kind regards Schneppat AI & GPT5 & Risikomanagement im Trading