Polynomial Regression: Modeling Complex Curvilinear Relationships
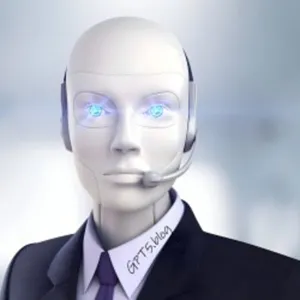
Polynomial Regression is a form of regression analysis in which the relationship between the independent variable x and the dependent variable y is modeled as an n th degree polynomial. Extending beyond the linear framework, polynomial regression is particularly adept at capturing the nuances of curvilinear relationships, making it a valuable tool in fields where the interaction between variables is inherently complex, such as in environmental science, economics, and engineering.
Understanding Polynomial Regression
At its essence, polynomial regression fits a nonlinear relationship between the value of x and the corresponding conditional mean of y, denoted E(y∣x), through a polynomial of degree n. Unlike linear regression that models a straight line, polynomial regression models a curved line, allowing for a more flexible analysis of datasets.
Key Features of Polynomial Regression
- Flexibility in Modeling: The ability to model data with varying degrees of curvature allows for a more accurate representation of the real-world relationships between variables.
- Degree Selection: The choice of the polynomial degree (n) is crucial. While a higher degree polynomial can fit the training data more closely, it also risks overfitting, where the model captures the noise along with the underlying relationship.
- Use Cases: Polynomial regression is widely used for trend analysis, econometric modeling, and in any scenario where the relationship between variables is known to be non-linear.
Advantages and Considerations
- Versatile Modeling: Can capture a wide range of relationships, including those where the effect of the independent variables on the dependent variable changes direction.
- Risk of Overfitting: Care must be taken to avoid overfitting by selecting an appropriate degree for the polynomial and possibly using regularization techniques.
- Computational Complexity: Higher degree polynomials increase the computational complexity of the model, which can be a consideration with large datasets or limited computational resources.
Applications of Polynomial Regression
Polynomial regression has broad applications across many disciplines. In finance, it can model the growth rate of investments; in meteorology, it can help in understanding the relationship between environmental factors; and in healthcare, it can be used to model disease progression rates over time.
Conclusion: A Powerful Extension of Linear Modeling
Polynomial Regression offers a powerful and flexible extension of linear regression, providing the means to accurately model and predict outcomes in scenarios where relationships between variables are non-linear. By judiciously selecting the polynomial degree and carefully managing the risk of overfitting, analysts and researchers can leverage polynomial regression to uncover deep insights into complex datasets across a variety of fields.
Kind regards Schneppat AI & GPT 5 & Geld- und Kapitalverwaltung