Scikit-Learn: Simplifying Machine Learning with Python
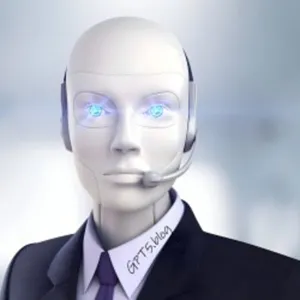
Scikit-learn is a free, open-source machine learning library for the Python programming language. Renowned for its simplicity and ease of use, scikit-learn provides a range of supervised learning and unsupervised learning algorithms via a consistent interface. It has become a cornerstone in the Python data science ecosystem, widely adopted for its robustness and versatility in handling various machine learning tasks. Developed initially by David Cournapeau as a Google Summer of Code project in 2007, scikit-learn is built upon the foundations of NumPy, SciPy, and matplotlib, making it a powerful tool for data mining and data analysis.
Core Features of Scikit-Learn
- Wide Range of Algorithms: Scikit-learn includes an extensive array of machine learning algorithms for classification, regression, clustering, dimensionality reduction, model selection, and preprocessing.
- Consistent API: The library offers a clean, uniform, and streamlined API across all types of models, making it accessible for beginners while ensuring efficiency for experienced users.
Challenges and Considerations
While scikit-learn is an excellent tool for many machine learning tasks, it has its limitations:
- Scalability: Designed for medium-sized data sets, scikit-learn may not be the best choice for handling very large data sets that require distributed computing.
- Deep Learning: The library focuses more on traditional machine learning algorithms and does not include deep learning models, which are better served by libraries like TensorFlow or PyTorch.
Conclusion: A Foundation of Python Machine Learning
Scikit-learn stands as a foundational library within the Python machine learning ecosystem, providing a comprehensive suite of tools for data mining and machine learning. Its balance of ease-of-use and robustness makes it an ideal choice for individuals and organizations looking to leverage machine learning to extract valuable insights from their data. As the field of machine learning continues to evolve, scikit-learn remains at the forefront, empowering users to keep pace with the latest advancements and applications.
See akso: Quantum Computing, Geld- und Kapitalverwaltung, Ethereum (ETH), SEO & Traffic News, Internet solutions ...
Kind regards Schneppat AI & GPT-5