Keras: Simplifying Deep Learning with a High-Level API
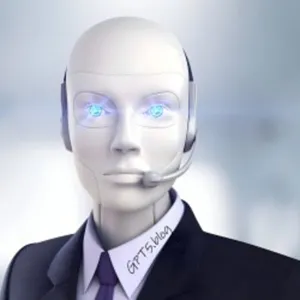
Keras is an open-source neural network library written in Python, designed to enable fast experimentation with deep learning algorithms. Conceived by François Chollet in 2015, Keras acts as an interface for the TensorFlow library, combining ease of use with flexibility and empowering users to construct, train, evaluate, and deploy machine learning (ML) models efficiently. Keras has gained widespread popularity in the AI community for its user-friendly approach to deep learning, offering a simplified, modular, and composable approach to model building and experimentation.
Applications of Keras
Keras has been employed in a myriad of applications across various domains, demonstrating its versatility and power:
- Video and Image Recognition: Leveraging convolutional neural networks (CNNs) for tasks such as image classification, object detection, and more.
- Natural Language Processing (NLP): Utilizing recurrent neural networks (RNNs) and transformers for language translation, sentiment analysis, and text generation.
- Generative Models: Creating generative adversarial networks (GANs) and variational autoencoders (VAEs) for image generation and more sophisticated generative tasks.
Advantages of Using Keras
- Ease of Use: Keras's API is intuitive and user-friendly, making it accessible to newcomers while also providing depth for expert users.
- Community and Support: Keras benefits from a large, active community, offering extensive resources, tutorials, and support.
- Integration with TensorFlow: Keras models can tap into TensorFlow's ecosystem, including advanced features for scalability, performance, and production deployment.
Conclusion: Accelerating Deep Learning Development
Keras stands out as a pivotal tool in the deep learning ecosystem, distinguished by its approachability, flexibility, and comprehensive functionality. By lowering the barrier to entry for deep learning, Keras has enabled a broader audience to innovate and contribute to the field, accelerating the development and application of AI technologies. Whether for academic research, industry applications, or hobbyist projects, Keras continues to be a leading choice for building and experimenting with neural networks.
Kind regards Schneppat AI & GPT 5 & SERP