Parametric Regression: A Foundational Approach to Predictive Modeling
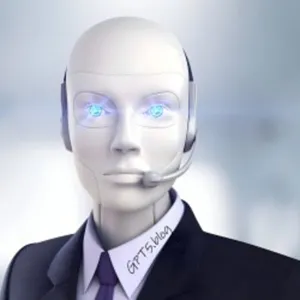
Parametric regression is a cornerstone of statistical analysis and machine learning, offering a structured framework for modeling and understanding the relationship between a dependent variable and one or more independent variables. This approach is characterized by its reliance on predefined mathematical forms to describe how variables are related, making it a powerful tool for prediction and inference across diverse fields, from economics to engineering.
Essential Principles of Parametric Regression
At its heart, parametric regression assumes that the relationship between the dependent and independent variables can be captured by a specific functional form, such as a linear equation in linear regression or a more complex equation in nonlinear regression models. The model parameters, representing the influence of independent variables on the dependent variable, are estimated from the data, typically using methods like Ordinary Least Squares (OLS) for linear models or Maximum Likelihood Estimation (MLE) for more complex models.
Common Types of Parametric Regression
- Simple Linear Regression (SLR): Models the relationship between two variables as a straight line, suitable for scenarios where the relationship is expected to be linear.
- Multiple Linear Regression (MLR): Extends SLR to include multiple independent variables, offering a more nuanced view of their combined effect on the dependent variable.
- Polynomial Regression: Introduces non-linearity by modeling the relationship as a polynomial, allowing for more flexible curve fitting.
- Logistic Regression: Used for binary dependent variables, modeling the log odds of the outcomes as a linear combination of independent variables.
Challenges and Considerations
- Model Misspecification: Choosing the wrong model form can lead to biased or inaccurate estimates and predictions.
- Assumptions: Parametric models come with assumptions (e.g., linearity, normality of errors) that, if violated, can compromise model validity.
Applications of Parametric Regression
Parametric regression's predictive accuracy and interpretability have made it a staple in fields as varied as finance, for risk assessment; public health, for disease risk modeling; marketing, for consumer behavior analysis; and environmental science, for impact assessment.
Conclusion: A Pillar of Predictive Analysis
Parametric regression remains a fundamental pillar of predictive analysis, offering a structured approach to deciphering complex relationships between variables. Its enduring relevance is underscored by its adaptability to a broad spectrum of research questions and its capacity to provide clear, actionable insights into the mechanisms driving observed phenomena.
Kind regards Schneppat AI & GPT-5 & Psychologie im Trading