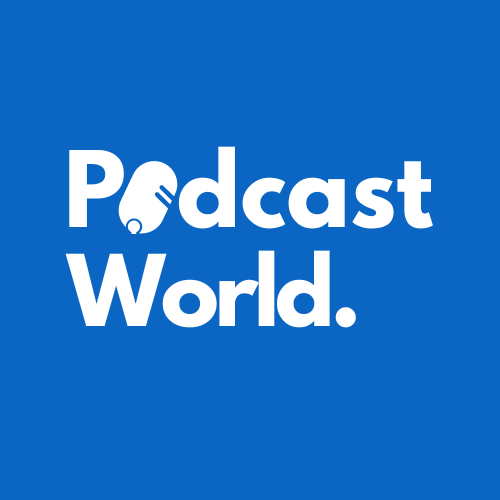
This proposal expands an understanding of humanitarian mapping from an ethnographic perspective, seeking to understand the complex mechanics behind this confluence of humanitarianism, technology, and crowdsourced labor. It seeks to scaffold a notion of the “open source mapping supply chain”, situating both humanitarian mapping and OpenStreetMap itself within a larger ecosystem of commercial, humanitarian, open source, government, and other actors in developing geospatial-related technologies.
This presentation presents a selection of a MA dissertation project, pursued over the course of more than 1.5 years of immersive fieldwork on OpenStreetMap. This presentation will focus on humanitarian mapping through qualitative study, seeking to expand an understanding of humanitarian mapping (particularly that which has emerged from mappers associated with the Humanitarian OpenStreetMap Team - also known as HOT) through the use of ethnographic tools, seeking to understand the complex mechanics behind this confluence of humanitarianism, technology, and crowdsourced labor, asking how and why people contribute to open-source platforms like OSM, and what role humanitarian mapping plays within the wider ecosystem of geospatial and mapping technologies. Ultimately however, it seeks to scaffold a notion of the “open source mapping supply chain”, situating both humanitarian mapping and OpenStreetMap itself within a larger ecosystem of commercial, humanitarian, open source, government, and other actors in developing geospatial-related technologies.
Founded in the aftermath of the 2010 earthquake in Haiti, the Humanitarian OpenStreetMap Team (HOT) helps both globally remote and local in-person volunteers to identify roads, buildings, and other features on the OpenStreetMap (OSM) platform. Created as a “free, editable map of the world,” OSM has enabled the mass-creation of volunteered geographical information (VGI) on a scale that is now more accurate than proprietary maps in many places, particularly as “crisis-mapping” has emerged as a means to gather real-time data on areas that have been affected by natural disasters or socio-political conflicts. OSM has also become also a site of resistance, where local and indigenous communities have engaged in mapping projects to reclaim autonomy, agency, and space through the historically contested practice of (digital) mapping. For these reasons, such crowdsourced maps have increasingly been used by humanitarian organisations to facilitate aid and disaster relief, and as open training data for algorithms learning how to automatically detect features through Artificial Intelligence (AI). As a key partner of humanitarian, corporate, and local actors, and having mobilised over 200,000 volunteers since 2010, HOT lies at the crux of these ongoing entanglements and contestations, both within and around the field of OSM.
Previous studies of crowdsourced geographical information and crisis-mapping have generally revolved around quantitative analyses of OSM’s data, focusing on the credibility of the data itself, the makeup of the communities that contribute to it, the effects of “event-centric” crowdsourcing, or “newcomer retention” in humanitarian mapping (Dittus et al., 2016a, 2016b, 2017; Haklay, 2010; Haworth et al., 2018; Sui et al., 2013). Alternatively, they have also focused on the “spatial knowledge”, “hacker political imaginary”, and gender composition of mappers themselves (Brandusescu & Sieber, 2018; McConchie, 2015; Stephens, 2013).
Parallel studies of other volunteer-driven communities like “Wikipedians” have taken similar approaches, analysing “user-generated content” and the motivations behind them (Nov, 2007; Yang & Lai, 2010). Both hacking and free and open source software (F/OSS) have also been explored ethnographically (Coleman, 2012; Kelty, 2008). While automated detection of features on OpenStreetMap has only recently become an important topic of research, ongoing studies have primarily focused on the accuracy or credibility of this endeavour (Brovelli et al., 2017; Resor, 2016).
While existing studies of digital communities have focused on the socialities they engender or labor they require, they tend to forget the bureaucratic apparatuses that have emerged to govern them, both implicitly and explicitly (Coleman, 2012; Kelty, 2008). Similarly, studies of humanitarianism have focused on the ethics they operationalize, or the technologies that are mobilized in turn, but often at the expense of engaging in the wider spectrum of social and economic life that they enable (Cross, 2013; Redfield, 2012, 2016a; Scott-Smith, 2013, 2016a, 2019; Ticktin, 2014a). While this project draws upon these overlapping strains of research, it seeks to push the debate in an ethnographic direction, scaffolded by theories of bureaucratic technology, political economy, and humanitarianism.
This research draws from participation in over 40 online events over 1.5 years, including mapathons, conferences and online lectures with OSM mappers, as well as semi-structured interviews conducted with 27 key-informants, alongside watching more conference videos, and reading blogs, mailing list emails, Twitter exchanges, and other internet archives. While empirically influenced by studies of hacking and open source software, this work ultimately focuses on the mechanisms and means through which this “free and open map” is created, and ultimately the ways of seeing and doing that it enables (Coleman, 2012; Kelty, 2008). Ultimately, it was the “supply chains” heuristic that emerged as a means to understand and illustrate this process.
Similar to how supply chains “link ostensibly independent entrepreneurs, making it possible for commodity processes to span the globe”, the OSM project relies upon a series interconnected processes that enable the creation of the world’s crowdsourced map in a process that is far more precarious, and much less secure than promotional material might have one think (Tsing, 2009). Similar to how the satellite, computer, and software industries converged to create the conditions that allowed for OSM’s creation, so do people – and their associated institutions create map data through an almost miraculous collision of circumstances, assured precedent, and training. The Humanitarian OpenStreetMap Team, which was the initial entry point into open source mapping through, made its name by optimizing the mapping value chain: that is, by making it easier to contribute to OSM. But it also extended outwards: contributing to OSM was enabled not only by the wider socio-economic forces that coalesced to produce the project in the first place, but also by a series of digital value chains – both past and present.
By delineating this supply-chains approach, this study hopes to scaffold a mental model of humanitarian mapping and the OpenStreetMap more broadly, to be employed in future studies – both quantitative and qualitative. Practically, it hopes to provide a heuristic and application of ethnographic tools, and present questions and queries directly to the community more broadly.
about this event: https://2022.stateofthemap.org/sessions/NWB9QF/